How Does Face Detection Work?
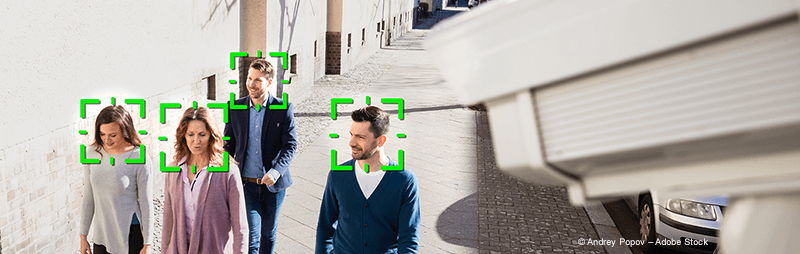
Face detection technology has come a long way over the past few years. From unlocking your iPhone by scanning your face to automatically tagging photographs, most of us have encountered and benefited from it (in one way or another).
However, there’s a lot more we can do with technology than just recognizing faces. For example, it is now a tool used in marketing to help improve sales and customer experiences. Or you can use this technology in offices to mark employee attendance or provide access to secure areas automatically. To understand the nuances of how online face recognition AI works and its applications, click here for an insightful read.
But before we get ahead of ourselves, let’s define it.
What Is Face Detection?
Face detection technology is exactly what it sounds like. It is a software that’s powered by artificial intelligence (AI) algorithms used to verify or identify a person’s identity. This is done by processing individual video frames or digital images where faces are visible.
In its infancy, we could only use facial detection software on a computer. Today, we can use this technology on mobile devices, robots, smart glasses, and more. This innovation led to an explosion of demand in industries like security and surveillance, marketing, and robotics.
How Do Face Detection Tools Work?
Face detection or facial recognition tools aren’t all the same. They use several different methods to compare facial features in an image and compare it to pictures stored in a database.
However, for the most part, face detection tools use intelligent algorithms that biometrically map out facial features captured in photographs and still video frames. Once the biometric map is complete, it is compared to an extensive database of faces. Understanding the variation and sophistication of these technologies can be vital for those seeking the cutting-edge in AI developments. For an in-depth exploration, click here to delve into the top facial recognition technologies.
Although there are many ways to do this, generally, we can detect faces in four simple steps:
Step 1: Face Recognition
First, a smart camera captures an image once it detects and recognizes a human face. This face can be in a crowd or some standing alone. This process is much easier when an individual looks directly at the camera. However, modern facial detection protocols still work when the person’s face is slightly angled.
Step 2: Face Analysis
Once an individual face is ready for analysis, the eye locations are determined. Then the image is automatically converted into grayscale and cropped. Most facial detection solutions available in the market today leverage 2D images instead of 3D to identify and verify individuals. This is because 2D photos are easier to correlate with images stored in databases (which are usually also 2D).
During the analysis, the tool separates the face into distinguishable landmarks known as nodal points. Every human face has eight nodal points, which AI-powered algorithms will analyze. For example, it might measure the distance between the eyes and compare it with other images in the database.
Step 3: Convert Images into Data
Upon conclusion of the facial analysis, each nodal point becomes a number that will be stored in the application database. All eight nodes together are referred to as a faceprint which is just like a unique thumbprint.
Step 4: Matching
Finding a facial match is the final step in the process. Sophisticated algorithms compare the recently created faceprint to other facial nodes stored in the database. The number of comparisons depends on the size of the database.
Whenever there’s a match, the facial detection application will display the match with other relevant information like the subject’s name, birth date, address, and so on. It’ll also return information such as the individual’s likes and dislikes, previous purchases, and more if it’s a commercial marketing database.
While the above describes the fundamental processes behind the technology, there are many other ways to achieve the same objective. For example, to improve accuracy, some tools project 2D images onto 3D models. This approach helps distinguish specific characteristics that would otherwise be difficult to detect in a flat 2D image.
Even though face detection technology is undoubtedly getting better, there are still some incorrect results. In particular, when older technological versions are used for face recognition, different errors can occur. These versions were mostly not trained with datasets that included sufficiently diverse ethnicities, ages, and genders. It is therefore important to rely on current tools that have been trained with a high diversity of data.
How to Optimize Face Detecting Tools
Your facial detection tool is only going to be as good as your AI training datasets. This is because AI and machine learning algorithms consistently get better as they are continuously exposed to more faces or images. If you want to minimize false positives and achieve more accuracy, it’ll help to expose smart algorithms to large datasets with people of different races, gender, and ages.
clickworker provides exactly these required training data sets. Have a look at the case study where clickworker provided a customer with training data sets to train a facial recognition tool that uses faces as biometric factors for online login and authentication protocols. For this purpose and aim, the tool’s algorithms are “fed” photos of thousands of Clickworkers spread across the planet.
Read the whole case study “Photo data sets for online face recognition training”
The commissioning of training data for the training of facial recognition technologies that can recognize emotions is also possible via clickworker. These technologies are sometimes already used in sales and marketing, helping sales teams to better engage customers in retail environments.
As the industry evolves, it’ll create multiple opportunities to boost revenue, security, and customer experiences. The future of face detection is incredibly promising!