Medical Data Annotation: The Key to Advancing Diagnostic AI
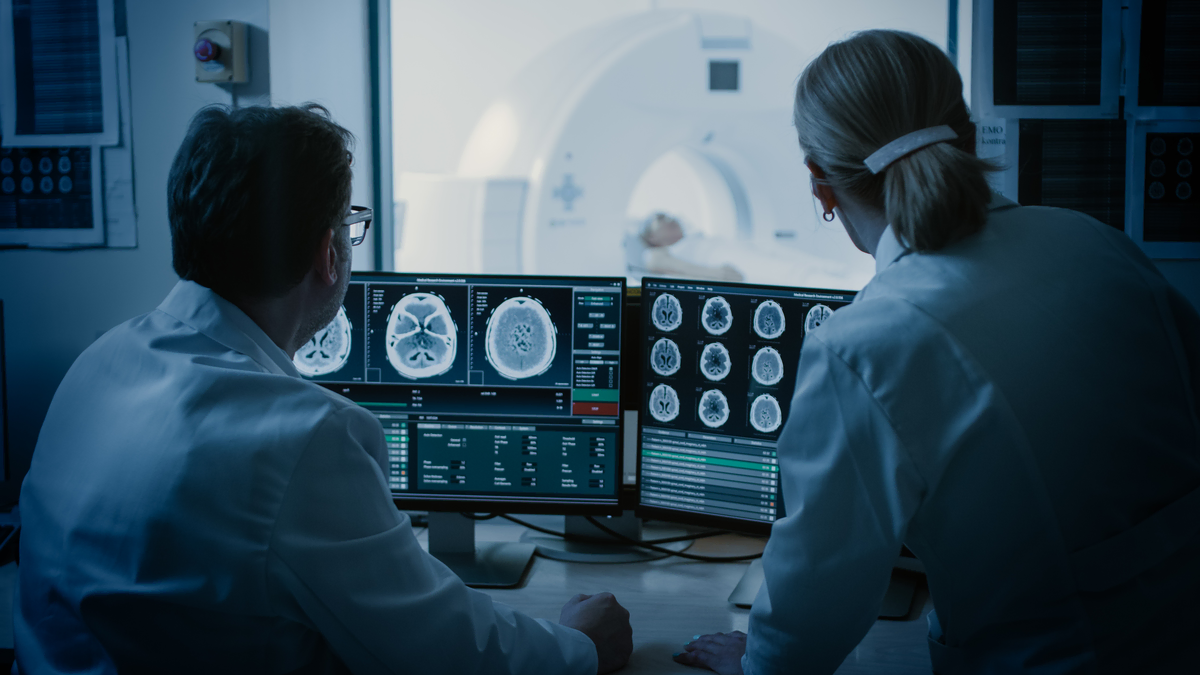
The global market for healthcare and medical data annotation tools is set to rise to $1.1 billion by 2032, showing an impressive annual growth rate of 23.85% from 2024 to 2032, according to the IMARC Group’s Healthcare Data Annotation Tools Market Report.
What’s driving this impressive growth? There are a few key reasons. First, we are seeing a surge in the use of artificial intelligence (AI) and machine learning (ML) in healthcare. Then there’s the fact that we’re generating huge amounts of data every day.
Additionally, there have been significant advancements in medical imaging technologies, along with improved access to high-quality image datasets.
The rising demand for telemedicine services highlights the importance of AI training data, which enhances diagnostic accuracy, personalized patient care, supports remote monitoring, and automates administrative tasks, ultimately improving the efficiency of remote healthcare delivery.
All these trends are driving the medical data annotation market forward, showing just how important these technologies are for improving healthcare delivery and patient outcomes.
Let’s look at why medical data annotation is important and how AI training data and image and photo datasets are transforming healthcare for the better.
Read moreLLM Hallucinations – Causes and Solutions
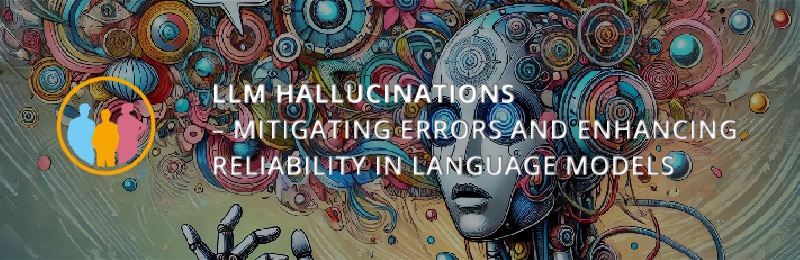
The precision and reliability of Artificial Intelligence (AI) are crucial, especially with large language models (LLMs). A common issue with these models is the phenomenon of “LLM hallucinations”. This term describes the tendency of language models to generate information not based on reality. This can range from incorrect facts to entirely fabricated stories.
LLM hallucinations pose a serious challenge as they can undermine the credibility and reliability of AI systems. They mainly arise due to insufficient or faulty training data, lack of contextualization, and the models’ excessive creativity. This problem affects both the developers of LLMs and businesses and end-users who rely on precise and reliable AI results.
To prevent these hallucinations and improve the quality of AI models, the provision of high-quality AI training data is crucial. This is where we, clickworker, come into play. We provide customized training data solutions and ensure the quality and accuracy of the data through crowdsourcing techniques and human review. By integrating these high-quality data, LLMs can work more precisely and reliably, leading to better results and increased user trust.
In this blog post, we will explore the causes and impacts of LLM hallucinations and show how clickworker’s services help address these challenges and improve the quality of language models.
Read moreWhy AI Agents Make AI More Practical and Useful
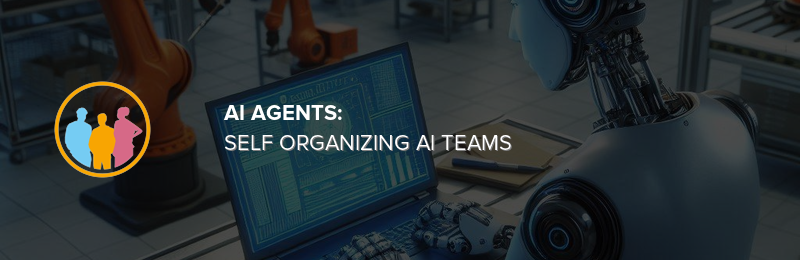
AI agents are intelligent entities that can operate autonomously, interact with other agents, and adapt to changing environments. This definition captures the key characteristics that make AI agents powerful and versatile tools in various applications.
The autonomy of AI agents allows them to perform tasks and make decisions without constant human intervention. They can process information, analyze situations, and take appropriate actions based on their programming and learned behaviors. This autonomous operation is crucial for handling complex tasks efficiently, especially in dynamic environments where real-time decision-making is essential.
Interaction is another vital aspect of AI agents. They can communicate and collaborate with other agents, whether artificial or human, to achieve common goals or solve complex problems. This ability to interact enables the creation of multi-agent systems where different specialized agents work together, leveraging their individual strengths to tackle challenges that would be difficult for a single agent to handle alone.
Read moreWhat is RAG (Retrieval Augmented Generation)?
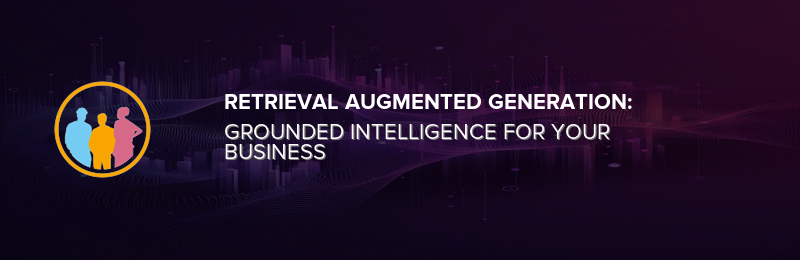
RAG (Retrieval-Augmented Generation) is an advanced AI architecture that combines the power of large language models (LLMs) with external knowledge retrieval. Here’s a breakdown of RAG, its workings, importance, and business applications:
You’re probably familiar with some of the shortcomings of current AI. For me, the most frustrating aspect is that you can’t rely on it for accurate information. Not only do current LLMs frequently ‘hallucinate’ facts, people, code libraries, events, and more – they state this information with such confidence that it can be hard to spot. This can be reduced with high quality training data, and fine tuning, but RAG is another powerful solution.
RAG is a hybrid AI system that enhances traditional language models by incorporating a retrieval step to fetch relevant information from external sources before generating responses. This approach allows AI to access up-to-date, factual information beyond its initial training data.
Read moreIs AI on the Path to Superintelligence?
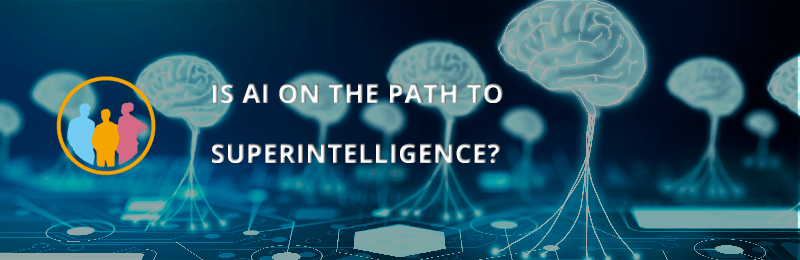
The rapid development in the field of artificial intelligence (AI) raises a crucial question: Will there ever be an AI superintelligence? The recent buzz around OpenAI and speculations about a mysterious project called “Q*” have reignited discussions about artificial general intelligence (AGI) and potential safeguards. Reports suggest that OpenAI has made progress in independently solving complex mathematical problems, which is seen as a step toward AGI. This has led to concerns and calls to slow down AI development and focus more on alignment with human values.
Regardless of specific advancements at OpenAI, the pace of AI development raises many fundamental questions. What is the current state of AGI research? What steps are necessary to get there? How do AGI and superintelligence differ? What ethical and societal implications arise from these developments? Experts shared their views and concerns on these topics during a virtual press briefing, emphasizing the importance of responsible and safe AI development.
Read moreA Milestone for Europe: The AI Act and Its Significance for Artificial Intelligence
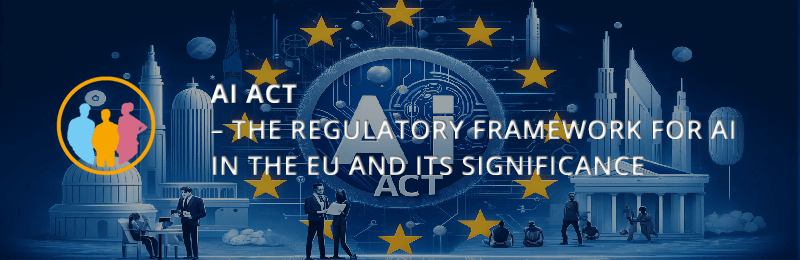
On May 21, 2024, the 27 EU member states adopted the AI Act, a comprehensive framework for regulating Artificial Intelligence (AI) within the European Union. This regulation is the world’s first comprehensive legal framework for AI, aiming to establish uniform standards and guidelines for the deployment of AI technologies. With the AI Act, the EU has laid a strong foundation for the regulation of artificial intelligence, promoting both trust and acceptance of the technology, as well as enabling innovations “made in Europe.”
The adoption of the AI Act by the EU Council is a significant step that will shape the future of artificial intelligence in Europe. The AI Act aims to maximize the benefits of AI while minimizing the risks. Through clear regulations and stringent requirements, it ensures that AI systems are deployed safely, transparently, and ethically. A crucial aspect of deploying AI ethically involves sentiment detection, which can help in understanding and navigating the intricacies of human emotions in digital communications.
In this blog post, we present the background and key contents of the AI Act, the specific provisions and their impact on innovation and the economy. We also highlight the national implementation in the member states and the international perspective of the AI Act.
Read moreThe Significance of Customized Speech Commands Datasets in AI Training Strategies
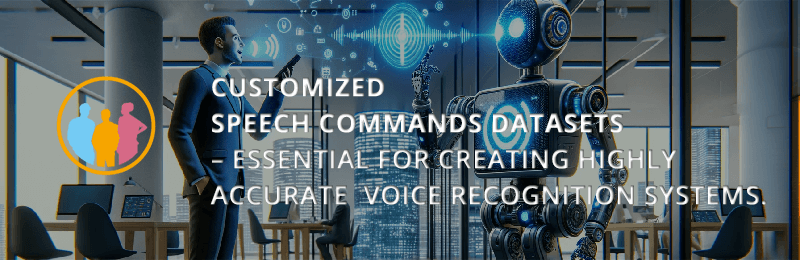
Have you noticed how AI is getting better at understanding us when we talk to our devices? It is all thanks to speech recognition technology. But to really make it work well, you as developers need to use customized speech commands datasets.
For example, think about when you are building a voice-controlled app. With a customized dataset, your app can understand specific commands better, like asking it to play a song or turn on the lights. It is like giving your app a superpower to understand fluent speech, context, and make the whole user experience smooth and intuitive.
For those seeking to enhance their speech recognition projects, exploring audio and voice datasets for speech recognition training can be a valuable resource.These datasets, tailored to specific applications and domains, are crucial in shaping the training strategies of AI systems, particularly in automatic speech recognition (ASR) and voice-controlled applications.
For those interested in deepening their understanding of how such datasets can be assembled and optimized, exploring resources like audio data collection could provide valuable insights. In this blog post, we will delve into the importance of using customized datasets designed for specific applications, and explore how personalized speech datasets contribute to more accurate, reliable, and context-aware AI models.
In this blog post, we will delve into the importance of using customized datasets designed for specific applications, and explore how personalized speech datasets contribute to more accurate, reliable, and context-aware AI models.
Read moreIncrease Your Productivity with AI Copilots
Welcome to the era of Generative Artificial Intelligence (Gen AI)! The buzz around this groundbreaking technology is contagious. It is accessible and gearing up to reshape organizations and the economy in ways that promise anything but dullness over the next decade.
According to McKinsey research, Gen AI is poised to automate 70% of business activities across various occupations by 2030, contributing trillions of dollars in value to the global economy.
Notably, the latest Gen AI application — AI Copilot, is garnering headlines for radically transforming the way businesses work amidst the complexities of digital modernization.
Much like digital Swiss Army knives, AI Copilots are adept at tasks ranging from boosting operational efficiency and aiding decision-making to fortifying security measures, simplifying content creation, and navigating intricate B2B sales processes.
Their versatility can be almost magical, leaving many intrigued about how to leverage this cutting-edge technology.
In this post, we’ll guide you through understanding AI Copilots and provide examples of how you can use them to unlock new levels of productivity and efficiency.
Read moreLLM Training: Strategies for Efficient Language Model Development
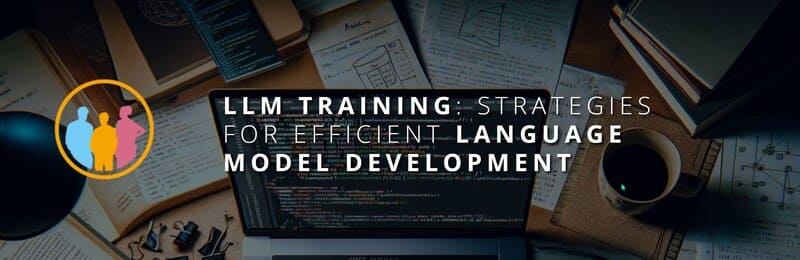
Content creation has been changed by large language models (LLM). These advanced machine learning architectures harness the power of vast amounts of textual data to perform a range of tasks under the umbrella of Natural Language Processing (NLP).
The training of LLMs involves meticulously structuring neural networks to generate human-like text, manage conversation, and even translate languages with remarkable accuracy.
Generative AI models, a subset of LLMs, are leading a paradigm shift in the way we interact with technology. Through training techniques that involve reinforcement from human feedback and innovations in model architectures, they have become central to developing AI systems that can comprehend and produce language effectively.
From streamlining customer service to powering virtual assistants, the applications of LLM are diverse, continuously expanding into new domains.
Their growing capabilities, however, come with a need for thoughtful consideration of ethical implications and the safety of AI systems. Ensuring that LLMs are trained to recognize and avoid harmful biases, respect user privacy, and make decisions transparently is critical for their responsible deployment.
Read moreHarnessing the Power of AI in Cybersecurity: The Future of Digital Defense
The evolution of cyber threats has called for an effective threat detection and prevention system in cybersecurity.
Enter AI.
Previously, cybersecurity used signature-based detection to identify threats and malicious activities. While effective, this system required the antivirus software to recognize the threat and it also relied significantly on manual analysis.
Machine learning algorithms have facilitated companies to detect new and unknown threats without the need for human intervention. One core aspect of these algorithms involves advanced text recognition techniques, which are pivotal in identifying and mitigating cyber threats with greater accuracy. AI has caused a major shift in how businesses approach cybersecurity and allowed them to look for advanced ways in which they can safeguard their data and systems.
Read more