Online face recognition: Face recognition with the help of AI
Author
Robert Koch
I write about AI, SEO, Tech, and Innovation. Led by curiosity, I stay ahead of AI advancements. I aim for clarity and understand the necessity of change, taking guidance from Shaw: 'Progress is impossible without change,' and living by Welch's words: 'Change before you have to'.
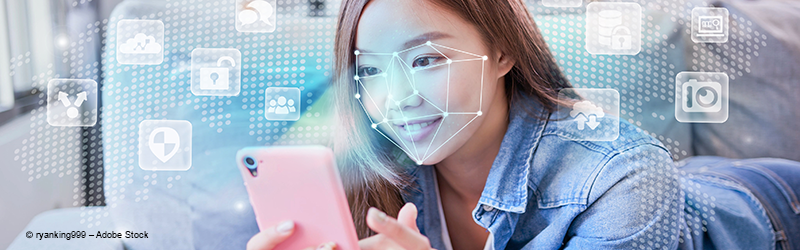
Identifying a face in images and videos is a standard task for artificial intelligence systems. Algorithms for online face recognition are trained based on millions of images obtained from the Internet and achieve increasingly reliable results. This not only applies to the assignment of image data to a specific person, but also to facial mood recognition and other relevant information for a wide range of applications. For a deeper dive into the technologies behind these advancements, explore the top 19 facial recognition technologies in detail.
Table of Contents
Introduction – facial recognition
Facial recognition, employs technology to visually map a person’s face and compare it to a database of recognized faces. Although this technology is widely used to confirm identities for personal purposes, its privacy consequences are still being investigated.
The usage of facial recognition technology is widespread and has many diverse applications. Smartphones with facial recognition technology as a way to access the device have been made available by companies like Apple, Samsung, and others. Facebook and Google use face recognition technology to automatically “tag” photographs and generate links. In addition to being utilized for social connections, advancements in deep learning and AI have significantly improved the accuracy of facial recognition systems, making these applications more efficient and reliable. The technology is increasingly being used by law enforcement for identifying offenders, with recent scrutiny over the FBI’s use for potential wrongful arrests and discrimination. Moreover, facial recognition is expanding into public spaces, such as malls and airports, to enhance security and efficiency, with notable examples including its implementation in the Mall of America. Airports, under collaborations with the Transportation Security Administration (TSA) and airlines like Delta and United, are adopting these systems to make travel smoother and more integrated.
Recent reports from the U.S. Commission on Civil Rights and the National Academies of Sciences, Engineering, and Medicine, alongside a growing body of research, have highlighted the need for federal regulations and guidelines on using facial recognition technology. These emphasize addressing civil rights implications, rigorously testing for fairness, and mitigating disparities across demographic groups. The global facial recognition market is on a rapid ascent, expected to grow from $5 billion in 2022 to $19 billion in 2032 at a CAGR of 14%, with approximately 70% of governments employing the technology significantly. This underscores the pressing importance of regulatory developments to ensure the ethical use of facial recognition technology amid rising ethical and privacy concerns, particularly regarding potential biases and inaccuracies affecting marginalized communities.
How does online face recognition work?
The human face is not static, it changes constantly. This variability of appearance is the most challenging aspect of automatic face recognition. In addition, some images are taken from different positions under constantly changing lighting conditions. People also have different hairstyles at different times – they wear makeup, glasses or hats.
An online face recognition program must therefore apply filters to identify the key recognition features. With deep learning, systems learn to make these distinctions on their own with the help of large amounts of data from the Internet. To achieve this, the systems work primarily with the recognition of patterns. The more data used to train them, and the higher quality that data is, the better the results of the AI algorithms.
Tip:
Thousands of Clickworkers create image datasets for the training of online face recognition algorithms.
Get more information about AI Training Datasets
Areas of application for online face recognition
There are numerous areas of application for online face recognition:
- Automated annotation of image files with meta-data for the systematic sorting of large data sets.
- Face recognition for access-sensitive areas.
- Authentication of mobile devices with the built-in camera.
A further example of the usefulness of face recognition and face identifier are mobile banking tools. In developing countries, the Aella Credit finance company provides simple identity verification for customers which functions without human intervention. This opens up the financial market to groups of people for whom it was previously inaccessible.
Online facial recognition is also a valid method for sensitive areas with access restrictions. Not only can existing systems compare image data of people with access rights, but in some cases they can also predict intentions based on typical features. AI systems for online face recognition can identify
- Eyes being open or closed,
- Visual geometry of a face,
- State of mind,
- Approximate age of an individual.
Informative video on applications of online face recognition
How Does Facial Recognition Work?
Facial recognition technology has evolved significantly since its inception. Initially, it was limited to 2D images, but advancements have led to the development of 3D facial recognition systems. These systems use various techniques to analyze and compare facial features.
2D Facial Recognition
2D facial recognition systems utilize images captured from a single perspective and identify key facial features in a 2D image. These features include:
- Distance between the eyes
- Nose width
- Jawline shape
These measurements are used to create a mathematical representation of the face for comparison.
Advantages of 2D Facial Recognition
- Generally less computationally expensive
- Can be used with widely available cameras
Limitations of 2D Facial Recognition2D facial recognition is susceptible to changes in:
- Lighting
- Pose
- Image quality
3D Facial Recognition Technologies
3D Morphable Face Models (3DMM)3DMM is a statistical model that uses principal component analysis on a large 3D facial dataset to generate a low-dimensional representation of face shape and texture. It is a technique used to create realistic 3D facial models and has applications in various fields like face recognition, animation, and even plastic surgery.
Feature ExtractionFeature extraction in 3D facial recognition involves identifying distinctive geometric features from the depth data. These features could include:
- Surface Normals: These vectors represent the orientation of a surface at a particular point. Analyzing the variation in surface normals provides information about the curves and contours of the face.
- Curvature: Curvature analysis helps in identifying prominent facial features like the tip of the nose, the chin, and the brow bone.
- Facial Landmarks: Identifying specific points on the face, like the corners of the eyes, the tip of the nose, and the corners of the mouth, provide a standardized way to compare different faces.
Deep LearningDeep learning has revolutionized 3D facial recognition by enabling the automatic learning of intricate patterns and representations from raw data. Convolutional Neural Networks (CNNs) are particularly effective in analyzing visual data like facial scans.
- Network Structure Optimization: Researchers are constantly working on improving the architecture of CNNs to enhance their ability to extract meaningful features from 3D facial data.
- Multi-Branch Structures: These networks process different aspects of the facial data (like color and depth) through separate branches and then fuse the information to make a more informed decision.
- Loss Function Engineering: Loss functions guide the training process of deep learning models. By designing specialized loss functions, researchers can train models that are more robust to variations in pose, illumination, and expression.
The Role of AI and Machine Learning in Accuracy and Bias Reduction
Training with Large DatasetsDeep learning models thrive on data. By training on massive and diverse datasets, these models can learn to generalize better and be less susceptible to biases. However, collecting high-quality, diverse 3D facial data is challenging and can be expensive.
Feature Extraction and RecognitionAI algorithms are capable of identifying subtle features that might be overlooked by traditional feature engineering methods. This improves accuracy, particularly in challenging conditions like varying lighting or facial expressions.
Bias Detection and MitigationAI can be used to analyze the performance of facial recognition models across different demographic groups. This analysis can reveal biases in the training data or the model itself. Once identified, steps can be taken to mitigate these biases. For instance, techniques like adversarial training can be used to make models more robust to variations in ethnicity or gender.
Regulatory Developments: The U.S. Commission on Civil Rights published a report in September 2024, addressing the civil rights implications of federal use of facial recognition technology, underscoring the need for accuracy, oversight, and transparency to prevent discrimination and ensure justice.
Global Use and Controversies: Facial recognition technology is utilized by approximately 70% of governments worldwide, sparking debates over privacy, civil rights, and its deployment in public spaces and law enforcement.
Market Growth: The market for facial recognition technology reached $5 billion USD in 2022, with projections suggesting a significant increase to $19 billion USD by 2032, indicating a compound annual growth rate of 14%.
The increasing role of AI and ML in creating more accurate, efficient, and potentially less biased facial recognition systems is evident. However, it is important to acknowledge the ethical implications and potential for misuse with this technology.
Face recognition optimization
Image recognition is now the main area of application for AI training in neural networks. Here, facial recognition plays a special role. Given the issues that artificial intelligence already has in classifying simple objects, it is clear that matching faces, which are often only minimally different, is particularly difficult. Furthermore, AI systems are susceptible to attack by cybercriminals. Even small changes to images can be used to provoke false results.
However, there are several ways to address these issues:
- Explainable AI exposes the structures that neural networks use as discriminators. This allows the rapid correction of potentially erroneous internal operations.
- Images specifically designed for cyber attacks are already being used in the training of AI systems. This helps detect corrupted image files more quickly.
- Training images should show faces from as many different positions as possible and also in unusual situations.
Many companies use the possibilities of crowdworking to create image files for training purposes. This provides them with customized AI training data set files that are tailored to their purposes within a short period of time.
Is online face recognition reliable?
The result of online face recognition is a probability value. Matching a person with an image is therefore only more or less reliable. However, the advantage of a machine-based similarity comparison is not only the fact that a program will usually provide the probability value at the same time. Research has shown that modern face identifier technologies are superior to the human eye. Google’s neural network Face Net, for example, achieves a hit rate of over 99 percent.
Various features determine the individuality of a face. These include the geometry of a face – i.e. the relationship between the eyes, the nose, the forehead and the mouth. A system designed to highlight similarities between a specific image and existing image data works with these key facial elements. The information regarding a person’s identity is superfluous as long as only similarities are searched for.
It is clear that automatic systems always provide only a probability value regarding matches, similarities, or differences. Depending on the areas of application, the user of the program can then select a similarity threshold that is sufficient for the purpose at hand. While facial recognition technologies have made significant strides in accuracy, recent studies have highlighted differences in performance across different demographic groups. For instance, NIST’s evaluations have shown that some algorithms exhibit higher false positive rates for certain racial and gender groups. In the public safety sector, a threshold of 99 percent is preferred, while for applications like online image search, a match probability as low as 95 percent is considered practical, though ongoing research and development aim to minimize these disparities.
Difference Between Face Identifier and Face Recognition
Facial technology has seen rapid advancements over the years, making the process of distinguishing between terms like “Face Identifier” and “Face Recognition” vital. While both are grounded in the science of analyzing facial features, their objectives and implementations differ. This chapter delves into these distinctions.
DefinitionBefore diving deeper into their differences, it’s essential to understand what each term means at its core.
- Face Identifier: This refers to a system or algorithm that assigns a unique identifier to each distinct face it detects. Essentially, it ‘labels’ or ‘tags’ faces but does not necessarily match them to known identities. Its main task is to differentiate one face from another.
- Face Recognition: This is a broader concept that encompasses the detection, analysis, and identification of faces against a database. It matches a detected face to a known identity in its database, thus ‘recognizing’ who the individual is.
Technological UnderpinningsThe technological backbone of these systems varies based on their core functionalities. Let’s explore the algorithms and mechanisms that drive them.
- Face Identifier
- Primarily relies on feature extraction to map unique facial features and assign a unique ID.
- Does not require a vast database of known faces, but rather a dynamic system that can generate and track unique identifiers.
- Face Recognition
- Employs deep learning algorithms, especially convolutional neural networks (CNNs), to detect and match facial patterns.
- Requires a database of known faces and their respective identities.
Privacy Implications
In today’s digital age, privacy concerns surrounding facial technologies have taken center stage. Understanding the privacy implications of each system is crucial for ethical deployment.
- Face Identifier
- Generally considered less intrusive, as it doesn’t tie facial data to real-world identities.
- Anonymizes data, which can be preferable in settings where user privacy is paramount.
- Face Recognition
- Raises more privacy concerns, given its ability to pinpoint identities.
- Usage often requires more stringent regulations and user consent.
Accuracy and ChallengesNo technology is without its hurdles. The efficacy of these facial systems, and the challenges they face, offer insight into their real-world applications.
- Face Identifier
- Main challenge lies in ensuring that the system consistently assigns the same identifier to an individual across various conditions.
- Face Recognition
- Accuracy can be impacted by factors like lighting, pose, and occlusions.
- Has been under scrutiny for potential biases, especially in recognizing diverse faces across races, ages, and genders.
Facial Recognition: Progress, Challenges, and RegulationFacial recognition technology has rapidly evolved, sparking both excitement and controversy. Initially adopted for security and convenience, it soon raised privacy concerns and accuracy issues, particularly affecting minorities. This led to pushback, with some jurisdictions restricting its use and companies reevaluating their offerings.
The EU’s Artificial Intelligence Act represents a significant regulatory effort. Key provisions include:
- Prohibition of real-time remote biometric identification in public spaces for law enforcement, with limited exceptions.
- Classification of many facial recognition applications as “high-risk” AI systems, requiring strict compliance and assessments.
- Allowance for member states to adopt stricter rules.
Critics argue the Act’s exceptions may still permit significant use in law enforcement and national security contexts. Despite challenges, researchers continue exploring positive applications in areas like assistive technology and healthcare diagnostics.
The path forward requires:
- Continued technological refinement
- Development of robust ethical frameworks
- Adaptive governance mechanisms
Balancing innovation with rights protection remains crucial. The ongoing dialogue between policymakers, technologists, and civil society is essential to harness benefits while mitigating risks, fostering responsible innovation in facial recognition technology.
Summary – online face recognition
Online face recognition using artificial intelligence offers numerous potential applications. However, the danger lies in using these automatic methods as an autonomous tool. Whenever personality rights are affected, additional human analysis must therefore be granted. In most areas, however, online face recognition is a powerful tool designed to make tasks easier and more efficient.
- Explainable AI exposes the structures that neural networks use as discriminators. This allows the rapid correction of potentially erroneous internal operations.
- Images specifically designed for cyber attacks are already being used in the training of AI systems. This helps detect corrupted image files more quickly.
- Training images should show faces from as many different positions as possible and also in unusual situations.
FAQs on Online Face Recognition
What is facial recognition?
Facial recognition is a piece of technology that can compare a human face in a digital photo or video frame to a database of faces.
How is facial recognition being used today?
The usage of facial recognition technology is widespread and has many diverse applications.
- Improving Security: Face recognition technology is used in surveillance cameras and even in unlocking your phone.
- Face recognition can be used in the medical field to identify diseases that result in physical changes that can be seen.
- Face recognition technology is employed in social media, where users can enhance their facial features by adding special effects or filters
What are the risks of online facial recognition?
- Identity theft and security
- Data security
- Violation of privacy